Comparative Analysis of Deep Learning Method Evaluation on Vehicle Type Classification
DOI:
https://doi.org/10.55732/jikdiskomvis.v9i`1.1252Keywords:
Convolutional Neural Network, Deep learning, ResNet50, VG16, Smart Transportation SystemAbstract
Vehicle recognition has high complexity, and problems that arise when conducting vehicle research such as variations in vehicle type, lighting conditions, perspective, resolution, image quality, and color and texture are the main factors. This problem requires a multidisciplinary approach with a combination of image processing technology, machine learning, and pattern recognition. Innovative approaches and research are consistently important to improve system performance as well as trying out all the deep learning architecture models that have been developed.
This research aims to compare Neural Network Models for class 1 to class 5 vehicle classification based on the type of classification on toll roads. The models compared in this research are Convolutional Neural Network, ResNet50, and VGG16. The model will be tested with input images of all parts of the vehicle after which the input images are resized to 224x224 for each input image. The scenario was carried out using 75 epochs in each model with a total of 500 data for each group and each group. The percentage of training data and test data is 80% training data and 20% test data. There are 5 class groups, namely Group 1, Group 2, Group 3, Group 4, Group 5.
The VGG16 model got the highest accuracy with 91% accuracy, Convolutional Neural Network 84% and ResNet50 got 74% accuracy. The results obtained show that the effectiveness of the VGG16 model is higher against CNN and ResNet50. Thus, this research can provide useful perceptions for further research to improve image capture with higher-quality cameras to improve intelligent transportation systems.
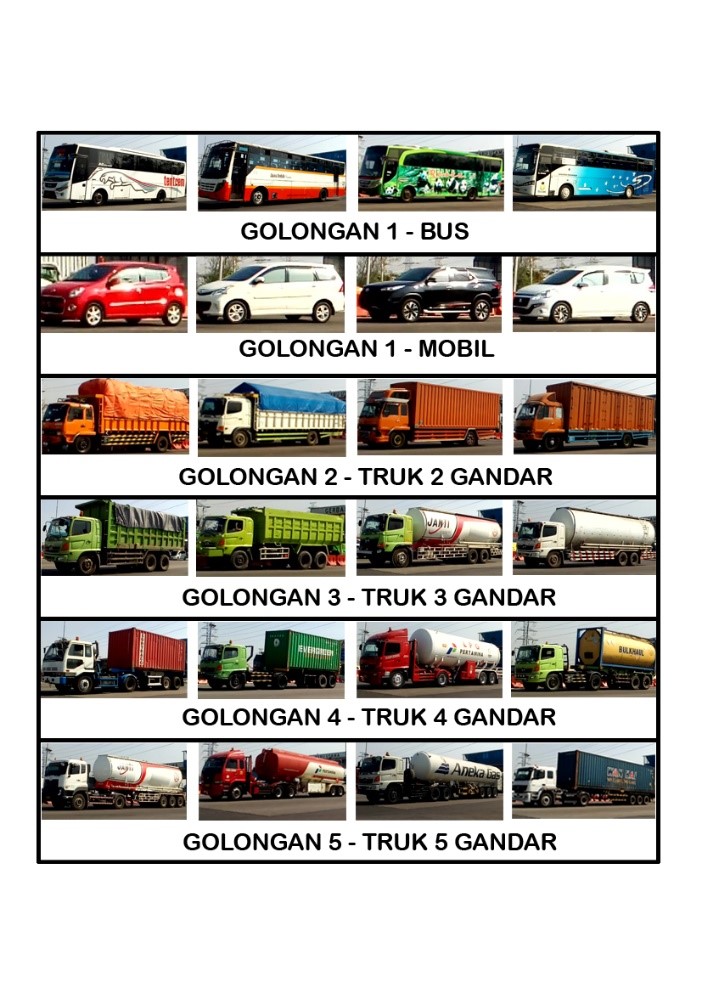
Downloads
Published
Issue
Section
License
Copyright (c) 2024 Journal of Computer Science and Visual Communication Design

This work is licensed under a Creative Commons Attribution-ShareAlike 4.0 International License.